Written by Laura Boykin, Simon Twigger, Brandon Patton and Christian Evans
Introduction
Watch the recording:
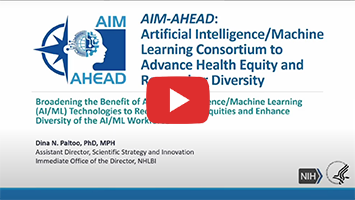
The artificial intelligence and machine learning (AI/ML) field currently lacks diversity in its researchers and in data, including electronic health record (EHR) data. These gaps pose a risk of creating and continuing harmful biases in how AI/ML is used, how algorithms are developed and trained, and how findings are interpreted. Critically, these gaps can lead to continued health disparities and inequities for underrepresented communities.
Underrepresented communities have untapped potential to contribute new expertise, data, recruitment strategies, and cutting-edge science to the AI/ML field. To close the gaps in the field and to better engage underrepresented communities, the National Institutes of Health (NIH) has launched the AI/ML Consortium to Advance Health Equity and Researcher Diversity (AIM-AHEAD) program.
This program seeks to increase the participation and representation of the researchers and communities that are currently underrepresented in AI/ML modeling and applications through mutually beneficial partnerships. AIM-AHEAD will also enhance AI/ML capabilities and has four key areas: partnerships, research, infrastructure and data science training.
The NIH hosted the AIM-AHEAD Stakeholder Engagement Forum on June 25, 2021 from 1-4 p.m. EDT with over 600 registrants and 374 attendees. The aim of this virtual forum was to bring together stakeholders across academia, federal agencies, the data science/tech industry, and health care systems and centers who are interested in leveraging AI/ML for research, with a primary focus on mitigating health disparities. The purpose of the forum was for the AIM-AHEAD team to hear from the stakeholders on ways to shape and sustain the AIM-AHEAD initiative into the future.
Summary
The meeting began with greetings from Lawrence Tabak, D.D.S., Ph.D., Principal Deputy Director, NIH followed by a brief overview of the initiative from Dina N. Paltoo, Ph.D., Assistant Director, Scientific Strategy and Innovation, Immediate Office of the Director, National Heart, Lung, and Blood Institute (NHLBI).
Two invited keynote speakers provided brief remarks to offer additional perspectives and stimulate further discussion in the subsequent breakout groups. Irene Dankwa-Mullan, M.D., Deputy Chief Health Officer and Chief Health Equity Officer, IBM Watson Health, IBM Corporation spoke about the “Potential of AI/ML for Health Disparities Research.” Her presentation was followed by remarks from Talitha M. Washington, Ph.D., Director, Data Science Initiative, Atlanta University Center Consortium, titled, “A Seat at the (Data) Table: Enhancing the Diversity of the AI/ML Workforce.”
At the conclusion of the keynote presentations, participants were placed in breakout groups based on their requested preferences: there were seven breakout groups on research and four each on training and infrastructure. The feedback from the various stakeholders was collected and is listed below.
Across all three breakout topics, two major themes emerged related to addressing disparities in AI/ML research, infrastructure, and training projects:
-
Ensure the team carrying out the research represents diverse voices and the community being served is fully represented throughout the entire duration of the project
-
A diverse and inclusive research team is important to ensure data sovereignty and data protection
These inclusive practices will build trust and ensure the reduction of bias in AI/ML research.
Nicole Redmond, M.D., Ph.D., Clinical Applications and Prevention Branch, Division of Cardiovascular Sciences, NHLBI, concluded the stakeholder forum by noting the emphasis on the importance of trust and how that plays into the quality of data, data security and privacy, and engagement in the AIM-AHEAD program overall.
Summary of Research Breakout Group Discussions
-
Take a community first approach: involve the community in building algorithms
-
Multi-level engagement of stakeholders in health care system is needed
-
Engage non-traditional stakeholders such as community health workers
-
Provide education for the public on AI/ML (bring algorithms out of the lab and make them available more publicly)
-
Involve Minority Serving Institutions (e.g., HBCUs, Tribal Colleges), Tribal Nations, and similar community organizations when conducting fundamental research
-
The greatest repositories of health disparity data and sources of human capacity to handle the threat of ML/AI sit with these groups and is being underutilized
-
These groups should be included at all levels of the research process. Partnerships need to be meaningful and not extractive.
-
Second class citizenship of the MSIs need to be addressed now and not later
-
"There is a role for AI/ML to help with identifying signatures in data, not just for the disease conditions but also to identify the resilience in the African-American populations." --Dr. Herman Taylor, Morehouse School of Medicine
-
Address trust building, data sovereignty, data protections and data inequities especially with Indigenous communities and scholars
-
Krystal Tsosie, Navajo geneticist and bioethics at Vanderbilt University, and colleagues have written several papers that should be considered by the wider community
-
Tsosie KS, Yracheta JM, Kolopenuk JA, Geary J. We Have "Gifted" Enough: Indigenous Genomic Data Sovereignty in Precision Medicine. Am J Bioeth. 2021 Apr;21(4):72-75. doi: 10.1080/15265161.2021.1891347. PMID: 33825628
-
Reconcile data types and structures of data capture
-
Address missing data, find a way to incorporate notes that provide context (e.g. EHR systems)
-
Social determinants of health are often not collected/missing
-
Detect/correct data biases
-
Enrich data sets with different types of data
-
Develop centralized data applications
-
Research partnerships between low and high resourced institutions must be clearly defined, equitable and inclusive. In addition, these research efforts must target community engagement at all levels.
-
NIH asks awardees to share data but more specific instructions/regulations should be given to encourage people to share data
Summary of Infrastructure Breakout Group Discussions
-
Infrastructure includes more than the hardware and software, it also includes people and social networks. Human resource infrastructure is tightly linked to training and building diverse and inclusive teams needed to reduce health disparities.
-
Develop uniform user-friendly AI/ML training materials to enable sharing with the community and onboarding of new team members
-
Improving equity—there is a need for more outreach and efforts for improving the pipeline to engage underrepresented groups
-
Cultural sensitivities must be considered when partnering with communities that may be under-resourced, marginalized, or rely on telemedicine; barriers in language and age
-
Lack of infrastructure at lower resourced community health centers biases the data being collected and used for decision making across the health sector. Suggestion is to invest in computation resources at these community health centers.
-
Hybrid compute infrastructure solutions (cloud and on-premise) to process AI/ML data ensures researchers from various resourced settings are able to benefit and contribute
-
Network capacity for sharing data is a concern
-
Internet access is not equal across the space so possible offline solutions need to be considered—especially for field locations where data are being collected and solutions are being provided
-
Infrastructure experts need to partner very early in the processes with domain experts and health disparities need to be highlighted at the inception to reduce bias in the data being collected
-
Historical data is not AI-ready and lacks race/ethnicity (black box data)
-
Social determinants of health are not incorporated into EHR (for example, using ZIP code to infer social economic status is riddled with bias)
-
Planning for infrastructure around AI/ML should also include data use agreements, governance and policy. Many spend years trying to get use agreements in place after the fact
-
Privacy needs to be considered and agreed upon by all partners
Summary of Training Breakout Broup Discussions
-
This is an interdisciplinary activity that includes researchers, physicians, data scientists, the community, and others. Engaging all of these groups and ensuring effective communication and collaboration between them will be essential to the success of this initiative.
-
It is important to acknowledge and address the knowledge and communication gaps between these groups that often stand in the way of bringing the field together. For example, ML scientists may need help communicating effectively with community members.
-
NIH should support Interdisciplinary training across the various perspectives
-
Address cultural awareness and address any structural barriers that may exist for certain groups
-
Retention of practicing physicians who can engage in ML/AI projects is challenging
-
It is hard for many of them to cross over to the area of ML/AI as physicians do not traditionally receive training in statistics and similar topics important to understand and contribute to ML/AI projects
-
Physician scientists need more support for training to fill in these gaps
-
Training
-
Audience
-
Introduce people to data very early on in their career, e.g. high school
-
Take active efforts to identify and engage underrepresented groups who should be included
-
Acknowledge and address the barriers that exist outside of research and training to ensure a diversity of people
-
Ensure people do not rule themselves out of ML/AI because they don't see themselves as being right type of person for the training program
-
Approaches
-
Indigi-data is a good example of inclusive training—took 10 years to build the connections and trust with indigenous people in genomics
-
Develop communities of practice, best practices for ML/AI
-
"Train the trainer" is an effective way to scale the provision of training
-
Partner with existing groups and look to industry for funding
-
The community should be engaged in what data is collected, how it is used and what questions need answering
-
Acknowledge that MSIs, etc., are not monolithic and that one size does not fit all
-
Career Development
-
Establish mentor/mentee relationships during the training programs
-
Sponsorship should be provided not just during training, but throughout career
-
Build trust and provide opportunities and incentives to get trainees actually employed the field
-
When people finish their training, what happens then? There is a need for incentives to keep people in the field, e.g. internship through industry.
-
Sustainability beyond the original program
-
It is important to ensure sustainability for programs established from this initiative so that they do not die once the original funding ends