Wednesday, January 31, 2024
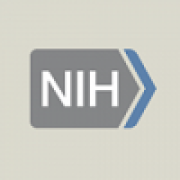
January 31–February 2, 2024
The goal of this workshop was to explore and assess the landscape of ethical AI in the biomedical research context by gathering expert input on opportunities and challenges with respect to the ethical use and reuse of data and models in the Artificial Intelligence (AI) development cycle. This interactive workshop was to:
- Begin to develop transparency guidelines for NIH awardees using, developing, or contributing to AI.
- Identify tools and capability gaps for ethical and transparent AI.
- Identify trends and future states.
This workshop was convened by the NIH Office of Data Science Strategy (ODSS) and the NIH-wide AI Ethics Working Group. ODSS leads implementation of the NIH Strategic Plan for Data Science through scientific, technical, and operational collaboration with the institutes, centers, and offices that comprise NIH.
Read the Co-Chair and Breakout Lead report of the workshop – including important opportunities to enhance transparency for researchers and others using, developing, or contributing to AI.
Agenda
Breakout Groups
In-person participants were given the choice of 5 different breakout themes to foster interactive discussions around key areas. Descriptions of each of the breakout sessions and their framing are outlined below.
Summaries
- Proxy Variables:
In this breakout session, we will examine the use of proxy variables in algorithms. Proxy variables are confounders and therefore are used (intentionally or unintentionally) in place of another variable that has a true causal relationship with the outcome. A notable example is the use of race and ethnicity in prediction models, as many experts believe that these variables are often oversimplified proxies for such variables as genetic ancestry or complex environmental and social factors. Other examples include the use of health care costs as a proxy for health care needs (Obermeyer 2019); given that less money is spent on Black patients who have the same level of need as White patients, the examined algorithm falsely concluded that Black patients are healthier than equally sick White patients. - Synthetic Data:
This breakout focuses on synthetic data—how they are generated, how they might be used, and how they could have both positive and negative impacts on human health. We will discuss specific considerations for the need for and challenges related to synthetic data, including realism, bias, degradation, ethical concerns, and generalizability. Where possible, specific examples will be discussed and used in developing best practices and guiding principles for ethical use and transparency of synthetic data.
Additional Resources:- Thorlund K, Dron L, Park JJH, Mills EJ. Synthetic and External Controls in Clinical Trials - A Primer for Researchers. Clin Epidemiol. 2020 May 8;12:457-467. doi: 10.2147/CLEP.S242097. PMID: 32440224; PMCID: PMC7218288.
- www.statnews.com/2019/02/05/synthetic-control-arms-clinical-trials/
- www.fda.gov/news-events/press-announcements/statement-fda-commissioner-scott-gottlieb-md-fdas-new-strategic-framework-advance-use-real-world
- nap.nationalacademies.org/catalog/26922/opportunities-and-challenges-for-digital-twins-in-biomedical-research-proceedings
- fastmri.org
- Multimodal Data
This breakout will discuss using multimodal data within artificial intelligence (AI) model development, validation, and translation for clinical implementation (e.g., combining structured data, such as diagnoses, with unstructured data, such as text or images). This will include specific considerations for the need for and challenges of generating and linking data in relation to ethics, bias, privacy, and transparency when combining complex, multimodal data with such details as time-course relevance.
Additional Resources:- HeLM: arxiv.org/pdf/2307.09018.pdf
- Multimodal wearables: Dunn, J., Kidzinski, L., Runge, R. et al. Wearable sensors enable personalized predictions of clinical laboratory measurements. Nat Med 27, 1105–1112 (2021).
doi.org/10.1038/s41591-021-01339-0
- Foundational Models:
Foundational models and the closely related Large Language Models (LLMs), such as ChatGPT, have sparked a huge wave of innovations combining the use of AI with the amazing capabilities of these models to integrate and deliver information. This breakout series will explore key concepts behind these models, as well as the implications when creating and using these models in multiple settings involving the clinician, patient, researcher, developer, and community as a whole. Anticipated areas of discussion include such topics as transparency, ethics, privacy, ownership, and reliability.
Additional Resources: - Data Sharing for General Reuse:
Data sharing has great potential to accelerate scientific innovation; however, it occurs without knowledge of how, whether, or by whom the data will be reused. Responsible reuse of shared data for AI requires technical, operational, ethical, privacy, and regulatory considerations to assess whether the data are fit for purpose.
Workshop Leads
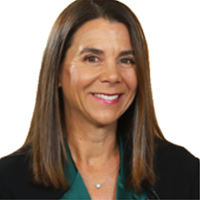
Tina Hernandez-Boussard, Ph.D., M.P.H., M.S. — Professor of Medicine
Stanford Medicine, Stanford University
Tina Hernandez-Boussard, Ph.D., M.P.H., M.S., is an Associate Dean of Research and Professor of Medicine (Biomedical Informatics), Biomedical Data Sciences, Surgery, and Epidemiology & Population Health (by courtesy) at Stanford University. Her background and expertise are in the field of biomedical informatics, health services research, and epidemiology. In her current work, Dr. Hernandez-Boussard develops and evaluates artificial intelligence (AI) technology to accurately and efficiently monitor, measure, and predict health care outcomes. She is a dedicated advocate of ethical AI practices.
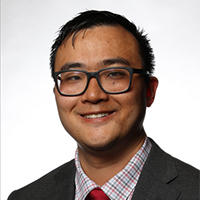
Aaron Y. Lee, M.D., M.Sci. — Associate Professor, University of Washington School of Medicine
Aaron Y. Lee M.D. MSCI is an associate professor and vitreoretinal surgeon at University of Washington, Department of Ophthalmology, and the recent recipient of the C. Dan and Irene Hunter Endowed Professorship. He completed his undergraduate at Harvard University and his medical training at Washington University in St Louis. He chairs the American Academy of Ophthalmology Information Technology Steering Committee. He currently serves as an Associate Editor for both Translational Vision Science and Technology and Ophthalmology Science, and on the Editorial Board for the American Journal of Ophthalmology and Nature Scientific Reports. He has published over 175 peer-reviewed manuscripts and is known as a leader in the field of artificial intelligence and ophthalmology. Aaron Lee' s research is focused on the translation of novel computation techniques in machine learning to uncover new disease associations and mechanisms from routine clinical data including electronic health records and imaging.
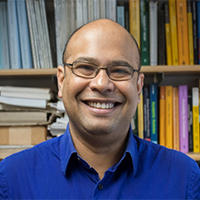
Ansu Chatterjee, Ph.D. — Sinha Ennovate Endowed Chair Professor, The University of Maryland Baltimore County
Dr. Snigdhansu (Ansu) Chatterjee is the Sinha Ennovate Endowed Chair Professor at the University of Maryland at Baltimore County. Until recently, he was a Professor in the School of Statistics at the University of Minnesota, and the Director of the Institute for Research in Statistics and its Applications (IRSA, http://irsa.stat.umn.edu/), an inter-disciplinary data science institute at the University of Minnesota, and a Data Scholar with the National Institutes of Health (NIH). His research interests include theoretical foundations of data sciences, high dimensional data geometry, Bayesian and other conditional inferential techniques, digital twins, selection bias, small samples in surveys and related topics, studies on ethics, fairness, diversity, privacy and representativeness in data sciences, and applications of data science techniques in multiple domains including precision medicine and climate change and its downstream effects.
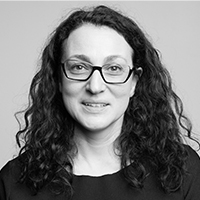
Julia Stoyanovich, Ph.D., M.S. — Institute Associate Professor, Tandon School of Engineering, New York University
Julia Stoyanovich, Ph.D., M.S., is Institute Associate Professor of Computer Science and Engineering, Associate Professor of Data Science, Director of the Center for Responsible AI, and member of the Visualization and Data Analytics Research Center at New York University. Dr. Stoyanovich’s goal is to make "Responsible AI" synonymous with "AI." She works toward this goal by engaging in academic research and education and technology policy, and by speaking to practitioners and members of the public about the benefits and harms of AI. Dr. Stoyanovich’s research interests include AI ethics and legal compliance, as well as data management and AI systems. In addition to academic publications, she has written for The New York Times, The Wall Street Journal, LA Times, The Hill, and Le Monde. Dr. Stoyanovich has been teaching courses on responsible data science and AI for students, practitioners, and the general public. She is a co-author of "Data, Responsibly," an award-winning comic book series for data science enthusiasts, and "We are AI," a comic book series for a general audience. Dr. Stoyanovich is engaged in technology policy and regulation in the United States and internationally, having served on the New York City Automated Decision Systems Task Force, by mayoral appointment, among other roles. She received her M.S. and Ph.D. in computer science from Columbia University and a B.S. in computer science and in mathematics & statistics from the University of Massachusetts Amherst. Dr. Stoyanovich’s work has been generously supported by the National Science Foundation (NSF), Pivotal Ventures, and Meta Responsible AI, among others. She is a recipient of the NSF CAREER Award and a Senior Member of the Association for Computing Machinery (ACM).
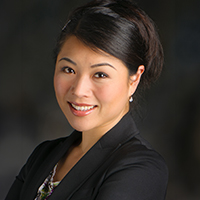
Caroline Chung, M.D., M.Sc., FRCPC, CIP — Vice President, Chief Data Officer
The University of Texas MD Anderson Cancer Center
Caroline Chung, M.D., M.Sc., FRCPC, CIP, is Vice President and Chief Data Officer and Director of Data Science Development and Implementation of the Institute of Data Science in Oncology at The University of Texas MD Anderson Cancer Center (MD Anderson). She is a clinician–scientist and an Associate Professor in Radiation Oncology and Diagnostic Imaging, with a clinical practice focused on central nervous system malignancies and a computational imaging lab focused on quantitative imaging and modeling to detect and characterize tumors and toxicities of treatment to enable personalized cancer treatment. Motivated by challenges observed in her own clinical and research pursuits, Dr. Chung has developed and leads institutional efforts to enable quantitative measurements for clinically impactful utilization and interpretation of data through a collaborative team science approach, including the Tumor Measurement Initiative (TMI) at MD Anderson.
Internationally, Dr. Chung leads several multidisciplinary efforts to improve the generation and utilization of high-quality quantitative data to drive research and impact clinical practice, including in her roles as Vice Chair of the Radiological Society of North America Quantitative Imaging Biomarker Alliance; Co-Chair of the Quantitative Imaging for Assessment of Response in Oncology Committee of the International Commission on Radiation Units and Measurements; and member of the National Academies of Sciences, Engineering, and Medicine–appointed committee addressing Foundational Research Gaps and Future Directions for Digital Twins. Beyond her clinical, research, and administrative roles, Dr. Chung enjoys serving as an active educator and mentor, with a passion for supporting the growth of diversity, equity, and inclusion in science, technology, engineering, and math, including through her role as Chair of Women in Cancer (www.womenincancer.com), a nonprofit organization that is committed to advancing cancer care by encouraging the growth, leadership, and connectivity of current and future oncologists, trainees, and medical researchers.
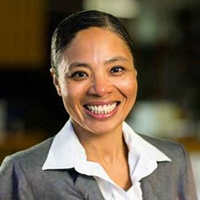
Maia Hightower, M.D., M.P.H., M.B.A. — Executive Vice President and Chief Digital Technology Officer, University of Chicago Medicine
Maia Hightower, M.D., M.P.H., M.B.A., is the CEO and co-founder of Equality AI and former Executive Vice President and Chief Digital Transformation Officer at University of Chicago Medicine. Dr. Hightower is a leading voice on the intersection of health care, digital transformation, and health equity. She is a champion for responsible AI, ensuring that the digital future of health care is equitable and just.
At the heart of Dr. Hightower’s mission is Equality AI, an early-stage, investor-backed health care tech startup. In a world where data scientists have become an integral part of the care team, Equality AI’s cloud-based, responsible AI life-cycle management platform—encompassing machine learning (ML) build, evaluate, monitor, and risk management—empowers health care with trustworthy AI, ensuring quality care for all.
She is a four-time C-suite physician executive with 15 years of executive leadership spanning health care IT, medical affairs, and population health across four academic medical centers, clinically integrated networks, and accountable care organizations.
Dr. Hightower received her B.A. from Cornell University and her M.D. and M.P.H. from the University of Rochester School of Medicine, followed by residencies in internal medicine and pediatrics at the University of California, San Diego. She also holds an M.B.A. from The Wharton School of the University of Pennsylvania.
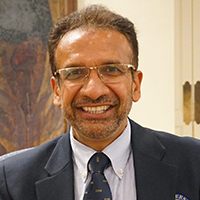
Sajid Hussain, Ph.D. — Associate Vice Provost for Innovation & Information Technology (CTO) and Discipline Coordinator of Data Science, Fisk University
Dr. Sajid Hussain is Associate Vice Provost for Research, Innovation, & Technology, Fisk University. In 2009, he joined Fisk University as an Associate Professor in the Department of Mathematics and Computer Science. Prior to Fisk, he worked as Associate Professor and Assistant Professor at Acadia University, Canada, 2005-09. He received a Ph.D. in Electrical Engineering from the University of Manitoba, Canada, in 2004.
Dr. Hussain is interested in applying machine learning techniques for interdisciplinary research projects related to healthcare and social justice. He is also interested in energy-efficient communication protocols and security techniques for mobile, ubiquitous, and pervasive applications. He has published more than 80 refereed journal, conference, and workshop papers. His research is financially supported by several grants and contracts, such as NSF INCLUDES National Data Science Alliance, NDSA (2217346), NSF Implementation Award (1817282), NSF IUSE (2235861), NIH/BD2K-R25 Diversity, Army, and Environment Management Department of Energy
He has co-organized several journal special issues, conferences, and workshops and is a senior member of IEEE.
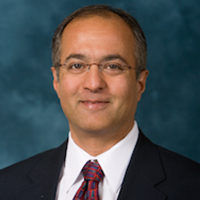
H.V. Jagadish, Ph.D. — Director, Michigan Institute for Data Science, University of Michigan
H.V. Jagadish, Ph.D., is the Edgar F. Codd Distinguished University Professor and Bernard A. Galler Collegiate Professor of Electrical Engineering and Computer Science at the University of Michigan in Ann Arbor and Director of the Michigan Institute for Data Science. Prior to 1999, he was Head of the Database Research Department at AT&T Labs in Florham Park, New Jersey.
Dr. Jagadish is well known for his broad-ranging research on information management and has more than 200 major papers and 38 patents, with an H-index of 101. He has been a fellow of the ACM, "The First Society in Computing," since 2003 and of the American Association for the Advancement of Science since 2018. He currently chairs the board of the Academic Data Science Alliance and previously served on the board of the Computing Research Association (2009–2018). He has been an Associate Editor for ACM Transactions on Database Systems (1992–1995), Program Chair of the ACM Special Interest Group on Management of Data (SIGMOD) annual conference (1996), Program Chair of the Intelligent Systems for Molecular Biology Conference (2005), a trustee of the VLDB (Very Large DataBase) foundation (2004–2009), Founding Editor-in-Chief of the Proceedings of the VLDB Endowment (2008–2014), and Program Chair of the VLDB Conference (2014). Since 2016, he has been the Editor of the Springer (previously Morgan & Claypool) Synthesis Lecture Series on Data Management. Among his many awards are the David E. Liddle Research Excellence Award (at the University of Michigan) in 2008, the ACM SIGMOD Contributions Award in 2013, and the Distinguished Faculty Achievement Award (at the University of Michigan) in 2019. His popular MOOC on Data Science Ethics is available on both EdX and Coursera.
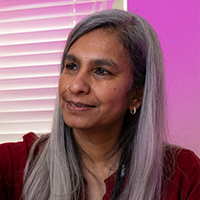
Jayashree Kalpathy-Cramer, Ph.D. — Chief of Artificial Medical Intelligence in Ophthalmology, University of Colorado School of Medicine
Jayashree Kalpathy-Cramer, Ph.D., is an Endowed Chair in Ophthalmic Data Sciences and the Founding Chief of the Division of Artificial Medical Intelligence in the Department of Ophthalmology at the University of Colorado School of Medicine. She leads the development and translation of novel AI methods into effective patient care practices at the Sue Anschutz-Rodgers Eye Center.
Previously, she was an Associate Professor of Radiology at Harvard Medical School, where she was actively involved in data science activities with a focus on medical imaging. Her research interests span the spectrum from novel algorithm development to clinical deployment. She is passionate about the potential that machine learning and mathematical modeling have to improve the quality of access to health care in the United States and worldwide. Dr. Kalpathy-Cramer has authored more than 250 peer-reviewed publications, has written more than a dozen book chapters, and is a co-inventor on a dozen patents. She graduated from the Indian Institute of Technology in Bombay, India, with a degree in electrical engineering and received her Ph.D. from Rensselaer Polytechnic Institute, also in electrical engineering. She returned to academia after almost a decade in the semiconductor industry, with a research pivot toward health care. Since then, her lab has been funded by the National Institutes of Health (NIH), National Science Foundation, and European Union.
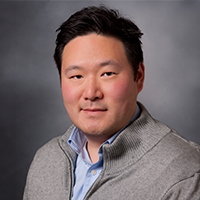
Vincent Liu, M.D., M.Sc. — Senior Research Scientist, Kaiser Permanente
Vincent Liu, M.D., M.Sc., is a Senior Research Scientist at the Kaiser Permanente (KP) Division of Research and the Regional Medical Director of the Hospital Advanced Analytics program at The Permanente Medical Group. He is an expert in the application of AI/ML methods to real-world health data and health care delivery, particularly for acute illness, including sepsis. He oversees the implementation of predictive analytics and AI/ML tools for a population of 4.5 million members in KP Northern California, the impact of which has been recognized through national and international awards for safety, innovation, and quality. He also leads the KP Augmented Intelligence in Medicine and Healthcare Initiative (KP AIM-HI), a $5 million program designed to support health systems to rigorously evaluate AI/ML implementation in diverse settings. He has co-authored 200 scholarly manuscripts in peer-reviewed journals and book chapters and has served as an expert advisor for NIH and the National Academy of Medicine, National Quality Forum, and National Committee on Quality Assurance. Dr. Liu maintains a clinical practice in pulmonary critical care medicine at the KP Santa Clara Medical Center and is the Informatics Track Director of the KP Division of Research Delivery Science Fellowship.
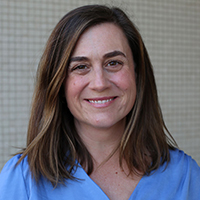
Courtney Lyles, Ph.D. — Director, Center for Healthcare Policy and Research, UC Davis Health
Courtney Lyles, Ph.D., is the Director of the UC Davis Health Center for Healthcare Policy and Research (CHPR) and Professor in the Department of Public Health Sciences in the UC Davis School of Medicine. A trained health services researcher, Dr. Lyles has expertise in health equity, digital health and informatics, and implementation science. Her research portfolio designs and evaluates new digital programs and platforms to support patients and families, as well as clinical workflows, with an emphasis on participant- and community-engaged methods and chronic disease prevention and treatment. She was a 2022–2023 Visiting Researcher at Google on its health equity team.
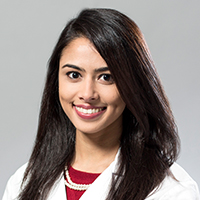
Shazia M. Siddique, M.D., M.S.H.P. — Assistant Professor in Gastroenterology
University of Pennsylvania Perelman School of Medicine
Shazia Siddique, M.D., M.S.H.P., is an Assistant Professor in Gastroenterology at the University of Pennsylvania Perelman School of Medicine. Dr. Siddique is an NIH-funded health services and health equity researcher. Her research, clinical expertise, and quality efforts aim to promote the integration of evidence-based practices into clinical care. She is the Associate Director of Scientific Research for the Center for Evidence-Based Practice at the University of Pennsylvania and serves on the Clinical Guidelines Committee for the American Gastroenterological Association. Additionally, she serves as Director for Research for the Penn Center for Healthcare Improvement and Patient Safety (CHIPS) program. Most recently, she was the senior author on the newly released report funded by the Agency for Healthcare Research and Quality’s Evidence-based Practice Center Program, titled "The Impact of Healthcare Algorithms on Racial and Ethnic Disparities in Health and Healthcare."
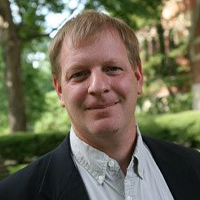
Eric Stahlberg, Ph.D. — Director, Cancer Data Science Initiatives, Frederick National Laboratory for Cancer Research
Eric Stahlberg, Ph.D., directs cancer data science initiatives at the Frederick National Laboratory for Cancer Research. He has been instrumental in establishing the Frederick National Laboratory’s high-performance computing (HPC) initiative and in assembling collaborative teams across multiple complex organizations to advance predictive oncology. Dr. Stahlberg has played a leadership role in many key partnerships, including forming the collaboration between the National Cancer Institute and the U.S. Department of Energy to accelerate progress in precision oncology and computing. Among the collaborative initiatives are IMPROVE (Innovative Methods and New Data for Predictive Oncology Model Evaluation), the Predictive Oncology Model and Data Clearinghouse (modac.cancer.gov), and ATOM (Accelerating Therapeutics for Opportunities in Medicine). He has led program efforts establishing foundations for digital twin applications in cancer, and now for advancing personalized medicine for all individuals through virtual human models and digital twin approaches. Among leading-edge initiatives, he also co-organizes the annual Computational Approaches for Cancer at SC conferences, the HPC Applications of Precision Medicine workshops at ISC, and cross-government workshops with the U.S. Food and Drug Administration. Most recently, he spearheaded the first Virtual Human Global Summit in October 2023, exploring the range of issues inherent in developing and appropriately employing the use of predictive health models and personal data within a digital twin approach.
Dr. Stahlberg has undergraduate degrees in chemistry, computer science, and mathematics and a Ph.D. in theoretical chemistry from The Ohio State University. He has been recognized as one of the FCW top 100, received the President’s Award from the Frederick National Laboratory for Cancer Research, and has received the distinguished alumni award from his alma mater, Wartburg College.
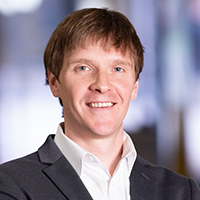
Colin G. Walsh, M.D., M.A., FAMIA, FACMI, FIAHSI — Associate Professor
Vanderbilt University Medical Center
Colin Walsh, M.D., M.A., FAMIA, FACMI, FIAHSI, is an Associate Professor of Biomedical Informatics, Medicine, and Psychiatry at the Vanderbilt University Medical Center. He is an internist whose research includes predictive decision support to enable prevention, scalable phenotyping for precision medicine, and population health informatics.
NIH Committee
Laura Biven, Ph.D.
Office of Data Science Strategy, National Institutes of Health
Susan Gregurick, Ph.D.
Associate Director for Data Science
Office of Data Science Strategy, National Institutes of Health
Christine Cutillo
Office of Data Science Strategy (ODSS)
Deborah Duran
National Institute on Minority Health and Health Disparities (NIMHD)
Samson Gebreab
Office of Data Science Strategy (ODSS), AIM-AHEAD
Christopher Kinsinger
Office of Strategic Coordination (OSC) – NIH Common Fund
Haluk Resat
Office of Strategic Coordination (OSC) – NIH Common Fund
David Resnik
National Institute of Environmental Health Sciences (NIEHS)
Asif Rizwan
National Heart, Lung, and Blood Institute (NHLBI)